Optimal non-uniform down-sampling
Body sensors are finding increasing applications in the self-monitoring for health-care and in the remote surveillance of sensitive people. The physiological data to be sampled can be non-stationary, with bursts of high amplitude and frequency content providing most information (e.g., ECG, EMG during gait, body acceleration during movement and possible falls). Such data could be sampled efficiently with a non-uniform schedule that increases the sampling rate only during activity bursts. This could be beneficial to lower the number of wireless communications (saving power in body sensor networks) and to reduce the occupation of memory. A real time and adaptive algorithm was developed to select the sampling rate, in order to reduce the number of measured samples, but still recording the main information. The algorithm is based on a neural network that predicts the subsequent samples and their uncertainties, requiring a measurement only when the risk of the prediction is larger than a selectable threshold. The method showed promising results in different applications (e.g., bioelectric signals, body acceleration), outperforming uniform sampling and compressive sensing in the accuracy of the reconstruction of data, with mean sampling rate far below the Nyquist limit. Moreover, when applied to heartbeats Holter monitoring, it was able to extract reliable information on heart rate variability and in the classification of different waveforms reflecting arrhythmias.
ERC Sector:
- PE7_7 Signal processing
Keywords:
- Nonuniform sampling
- Body sensor networks
Gruppi di ricerca
Persone di riferimento
- MESIN LUCA - Responsabile
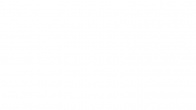
